Resolving Ambiguities in Text-to-Image Generative Models
Ninareh Mehrabi, Palash Goyal, Apurv Verma, Jwala Dhamala, Varun Kumar, Qian Hu, Kai-Wei Chang, Richard Zemel, Aram Galstyan, and Rahul Gupta, in ACL, 2023.
Download the full text
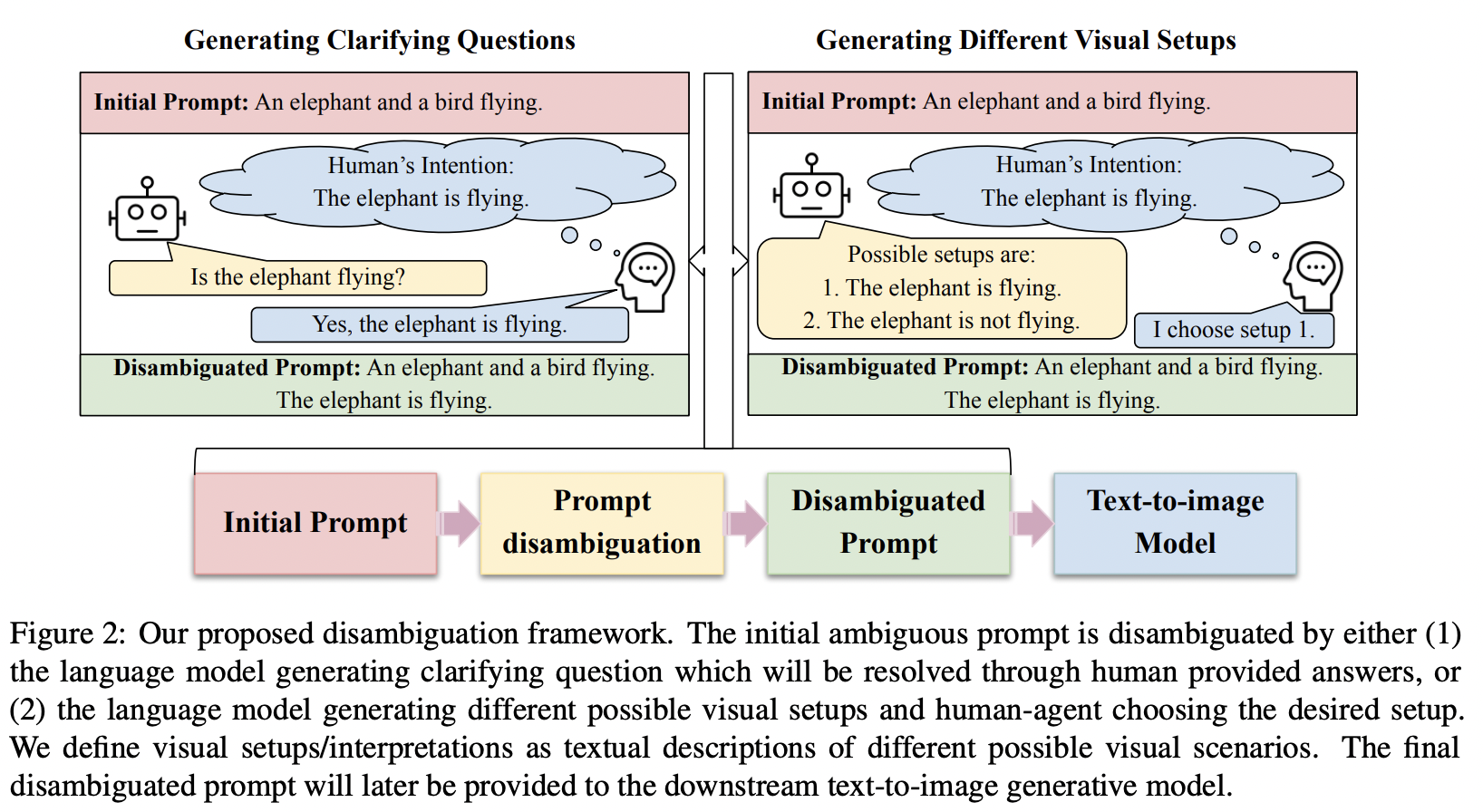
Abstract
Natural language often contains ambiguities that can lead to misinterpretation and miscommunication. While humans can handle ambiguities effectively by asking clarifying questions and/or relying on contextual cues and common-sense knowledge, resolving ambiguities can be notoriously hard for machines. In this work, we study ambiguities that arise in text-to-image generative models. We curate a benchmark dataset covering different types of ambiguities that occur in these systems. We then propose a framework to mitigate ambiguities in the prompts given to the systems by soliciting clarifications from the user. Through automatic and human evaluations, we show the effectiveness of our framework in generating more faithful images aligned with human intention in the presence of ambiguities.
Bib Entry
@inproceedings{mehrabi2023resolving, author = {Mehrabi, Ninareh and Goyal, Palash and Verma, Apurv and Dhamala, Jwala and Kumar, Varun and Hu, Qian and Chang, Kai-Wei and Zemel, Richard and Galstyan, Aram and Gupta, Rahul}, booktitle = {ACL}, title = {Resolving Ambiguities in Text-to-Image Generative Models}, presentation_id = {https://underline.io/events/395/posters/15237/poster/76575-resolving-ambiguities-in-text-to-image-generative-models}, year = {2023} }