PIP: Parse-Instructed Prefix for Syntactically Controlled Paraphrase Generation
Yixin Wan, Kuan-Hao Huang, and Kai-Wei Chang, in ACL-Finding (short), 2023.
Download the full text
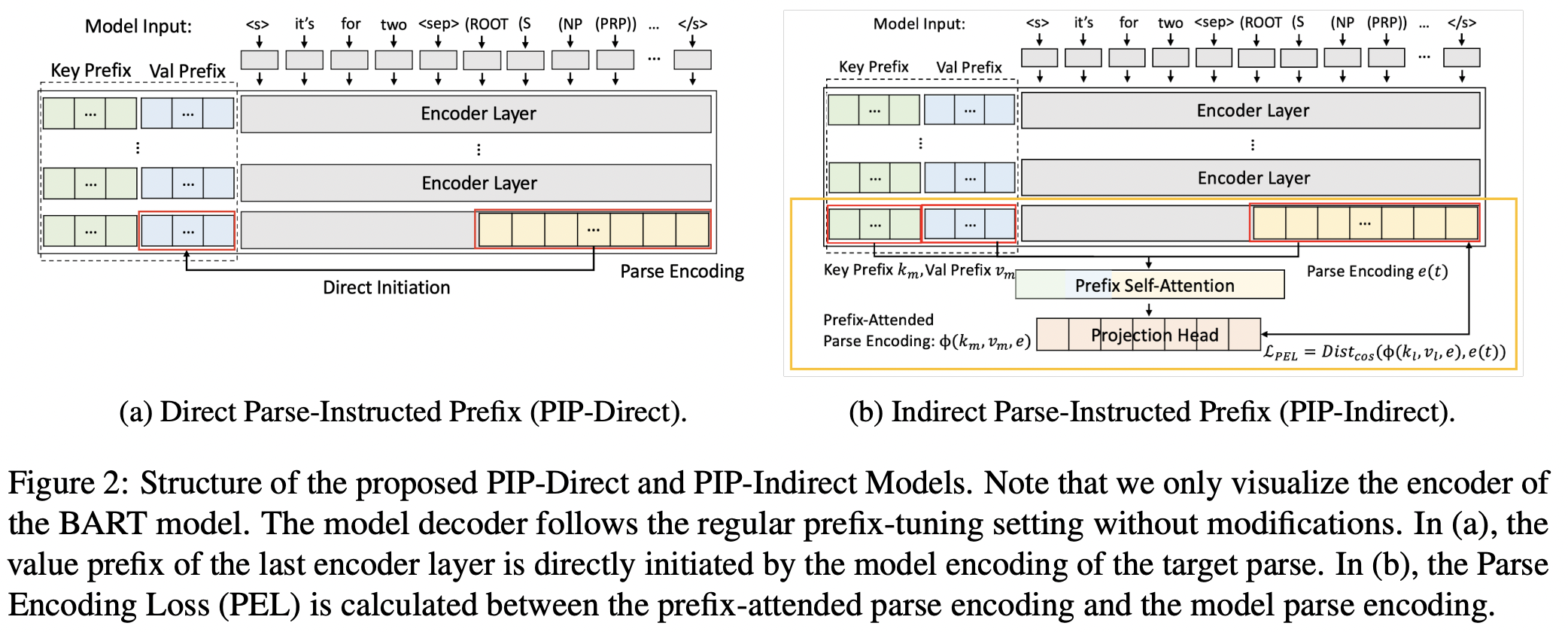
Abstract
Syntactically controlled paraphrase generation requires language models to generate paraphrases for sentences according to specific syntactic structures. Existing fine-tuning methods for this task are costly as all the parameters of the model need to be updated during the training process. Inspired by recent studies on parameter-efficient learning, we propose Parse-Instructed Prefix (PIP), a novel adaptation of prefix-tuning to tune large pre-trained language models on syntactically controlled paraphrase generation task in a low-data setting with significantly less training cost. We introduce two methods to instruct a model’s encoder prefix to capture syntax-related knowledge: direct initiation (PIP-Direct) and indirect optimization (PIP-Indirect). In contrast to traditional fine-tuning methods for this task, PIP is a compute-efficient alternative with 10 times less learnable parameters. Compared to existing prefix-tuning methods, PIP excels at capturing syntax control information, achieving significantly higher performance at the same level of learnable parameter count.
Bib Entry
@inproceedings{wan2023pip, author = {Wan, Yixin and Huang, Kuan-Hao and Chang, Kai-Wei}, title = {PIP: Parse-Instructed Prefix for Syntactically Controlled Paraphrase Generation}, booktitle = {ACL-Finding (short)}, presentation_id = {https://underline.io/events/395/posters/15279/poster/77944-pip-parse-instructed-prefix-for-syntactically-controlled-paraphrase-generation}, year = {2023} }