VisualBERT: A Simple and Performant Baseline for Vision and Language
Liunian Harold Li, Mark Yatskar, Da Yin, Cho-Jui Hsieh, and Kai-Wei Chang, in Arxiv, 2019.
CodeDownload the full text
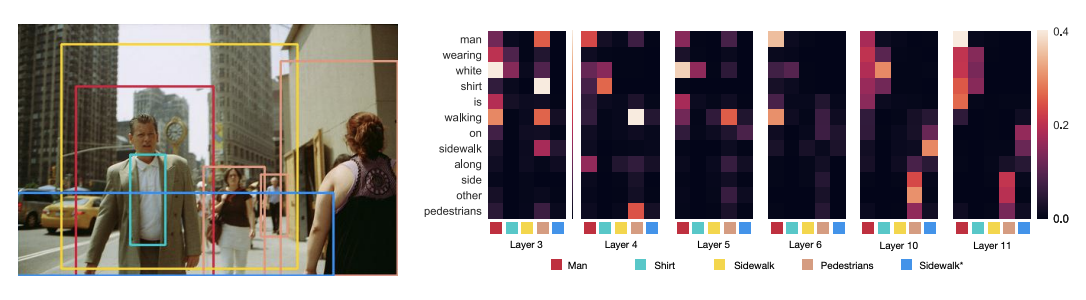
Abstract
We propose VisualBERT, a simple and flexible framework for modeling a broad range of vision-and-language tasks. VisualBERT consists of a stack of Transformer layers that implicitly align elements of an input text and regions in an associated input image with self-attention. We further propose two visually-grounded language model objectives for pre-training VisualBERT on image caption data. Experiments on four vision-and-language tasks including VQA, VCR, NLVR2, and Flickr30K show that VisualBERT outperforms or rivals with state-of-the-art models while being significantly simpler. Further analysis demonstrates that VisualBERT can ground elements of language to image regions without any explicit supervision and is even sensitive to syntactic relationships, tracking, for example, associations between verbs and image regions corresponding to their arguments.
hot off the press -- VisualBert: A simple and performant baseline for vision and language. Language + image region proposals -> stack of Transformers + pretrain on captions = SOTA or near on 4 V&L problems. https://t.co/uQ4O2Jhe2S @LiLiunian +Cho-Jui Hsieh +Da Yin @kaiwei_chang
— Mark Yatskar (@yatskar) August 12, 2019
VisualBERT is intergrated into Facebook MMF library
Please see more anlaysis about VisualBERT in a recent paper by A. Singh, V. Goswami, V., and D. Parikh (2019)
VisualBERT is used as a baseline in Hateful Memes by Facebook Research
Bib Entry
@inproceedings{li2019visualbert, author = {Li, Liunian Harold and Yatskar, Mark and Yin, Da and Hsieh, Cho-Jui and Chang, Kai-Wei}, title = {VisualBERT: A Simple and Performant Baseline for Vision and Language}, booktitle = {Arxiv}, year = {2019} }
Related Publications
-
Broaden the Vision: Geo-Diverse Visual Commonsense Reasoning
Da Yin, Liunian Harold Li, Ziniu Hu, Nanyun Peng, and Kai-Wei Chang, in EMNLP, 2021.
Full Text Code Abstract BibTeX DetailsCommonsense is defined as the knowledge that is shared by everyone. However, certain types of commonsense knowledge are correlated with culture and geographic locations and they are only shared locally. For example, the scenarios of wedding ceremonies vary across regions due to different customs influenced by historical and religious factors. Such regional characteristics, however, are generally omitted in prior work. In this paper, we construct a Geo-Diverse Visual Commonsense Reasoning dataset (GD-VCR) to test vision-and-language models’ ability to understand cultural and geo-location-specific commonsense. In particular, we study two state-of-the-art Vision-and-Language models, VisualBERT and ViLBERT trained on VCR, a standard multimodal commonsense benchmark with images primarily from Western regions. We then evaluate how well the trained models can generalize to answering the questions in GD-VCR. We find that the performance of both models for non-Western regions including East Asia, South Asia, and Africa is significantly lower than that for Western region. We analyze the reasons behind the performance disparity and find that the performance gap is larger on QA pairs that: 1) are concerned with culture-related scenarios, e.g., weddings, religious activities, and festivals; 2) require high-level geo-diverse commonsense reasoning rather than low-order perception and recognition.
@inproceedings{yin2021broaden, title = { Broaden the Vision: Geo-Diverse Visual Commonsense Reasoning}, author = {Yin, Da and Li, Liunian Harold and Hu, Ziniu and Peng, Nanyun and Chang, Kai-Wei}, booktitle = {EMNLP}, presentation_id = {https://underline.io/events/192/sessions/7790/lecture/37514-broaden-the-vision-geo-diverse-visual-commonsense-reasoning}, year = {2021} }
-
Unsupervised Vision-and-Language Pre-training Without Parallel Images and Captions
Liunian Harold Li, Haoxuan You, Zhecan Wang, Alireza Zareian, Shih-Fu Chang, and Kai-Wei Chang, in NAACL, 2021.
Full Text Video Abstract BibTeX DetailsPre-trained contextual vision-and-language (V&L) models have brought impressive performance improvement on various benchmarks. However, the paired text-image data required for pre-training are hard to collect and scale up. We investigate if a strong V&L representation model can be learned without text-image pairs. We propose Weakly-supervised VisualBERT with the key idea of conducting "mask-and-predict" pre-training on language-only and image-only corpora. Additionally, we introduce the object tags detected by an object recognition model as anchor points to bridge two modalities. Evaluation on four V&L benchmarks shows that Weakly-supervised VisualBERT achieves similar performance with a model pre-trained with paired data. Besides, pre-training on more image-only data further improves a model that already has access to aligned data, suggesting the possibility of utilizing billions of raw images available to enhance V&L models.
@inproceedings{li2021unsupervised, author = {Li, Liunian Harold and You, Haoxuan and Wang, Zhecan and Zareian, Alireza and Chang, Shih-Fu and Chang, Kai-Wei}, title = {Unsupervised Vision-and-Language Pre-training Without Parallel Images and Captions}, booktitle = {NAACL}, presentation_id = {https://underline.io/events/122/sessions/4269/lecture/19725-unsupervised-vision-and-language-pre-training-without-parallel-images-and-captions}, year = {2021} }
-
What Does BERT with Vision Look At?
Liunian Harold Li, Mark Yatskar, Da Yin, Cho-Jui Hsieh, and Kai-Wei Chang, in ACL (short), 2020.
Full Text Slides Video Code Abstract BibTeX DetailsPre-trained visually grounded language models such as ViLBERT, LXMERT, and UNITER have achieved significant performance improvement on vision-and-language tasks but what they learn during pre-training remains unclear. In this work, we demonstrate that certain attention heads of a visually grounded language model actively ground elements of language to image regions. Specifically, some heads can map entities to image regions, performing the task known as entity grounding. Some heads can even detect the syntactic relations between non-entity words and image regions, tracking, for example, associations between verbs and regions corresponding to their arguments. We denote this ability as \emphsyntactic grounding. We verify grounding both quantitatively and qualitatively, using Flickr30K Entities as a testbed.
@inproceedings{li2020what, author = {Li, Liunian Harold and Yatskar, Mark and Yin, Da and Hsieh, Cho-Jui and Chang, Kai-Wei}, title = {What Does BERT with Vision Look At?}, booktitle = {ACL (short)}, presentation_id = {https://virtual.acl2020.org/paper_main.469.html}, year = {2020} }
-
VisualBERT: A Simple and Performant Baseline for Vision and Language
Liunian Harold Li, Mark Yatskar, Da Yin, Cho-Jui Hsieh, and Kai-Wei Chang, in Arxiv, 2019.
Full Text Code Abstract BibTeX DetailsWe propose VisualBERT, a simple and flexible framework for modeling a broad range of vision-and-language tasks. VisualBERT consists of a stack of Transformer layers that implicitly align elements of an input text and regions in an associated input image with self-attention. We further propose two visually-grounded language model objectives for pre-training VisualBERT on image caption data. Experiments on four vision-and-language tasks including VQA, VCR, NLVR2, and Flickr30K show that VisualBERT outperforms or rivals with state-of-the-art models while being significantly simpler. Further analysis demonstrates that VisualBERT can ground elements of language to image regions without any explicit supervision and is even sensitive to syntactic relationships, tracking, for example, associations between verbs and image regions corresponding to their arguments.
@inproceedings{li2019visualbert, author = {Li, Liunian Harold and Yatskar, Mark and Yin, Da and Hsieh, Cho-Jui and Chang, Kai-Wei}, title = {VisualBERT: A Simple and Performant Baseline for Vision and Language}, booktitle = {Arxiv}, year = {2019} }