Datasets: CAMPUS Multi-view Object Tracking Dataset
General Introduction
We propose a hierarchical composition approach for multi-view object tracking. The key idea is to adaptively exploit multiple cues in both 2D and 3D, e.g., ground occupancy consistency, appearance similarity, motion coherence etc., which are mutually complementary while tracking the humans of interests over time.
- We define a set of composition criteria. Each criterion focuses on utilizing one certain cue.
- We learn parameters of each criteria separately by gradient descent and infer the compositional structure by a greedy pursuit algorithm.
- We establish a new dataset to provide richer information and cover more challenges in multi-view people tracking.
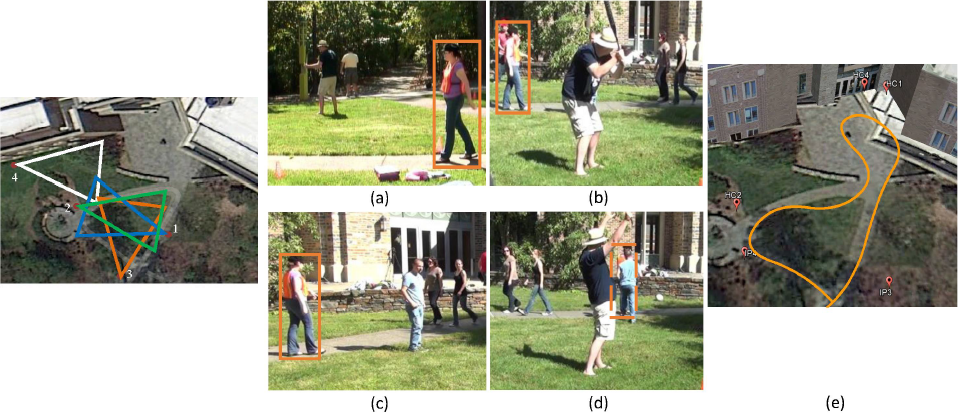
Dataset Overview
We collect this new dataset targeted for multi-view object tracking algorithms to test on dense foreground, complex scenarios, various object scales.
- Dense foreground: around 15-25 objects, frequent conjunctions and occlusions.
- Complex scenarios: objects conducting diverse activities, dynamic background, interactions between objects and background.
- Various object scales: tracking targets sometimes either too tiny or huge to be accommodated in certain cameras.
There are 4 sequences in this dataset: Garden 1, Garden 2, Auditorium and Parking Lot. Each sequence is shot by 3-4 high-quality DV cameras mounted around 1.5m-2m above ground and each camera covers both overlapping regions and non-overlapping regions with other cameras.
The videos are recorded with frame rate 30fps and duration about 3-4 minutes. The resolution is preserved in 1920X1080, for better precision and richer information.
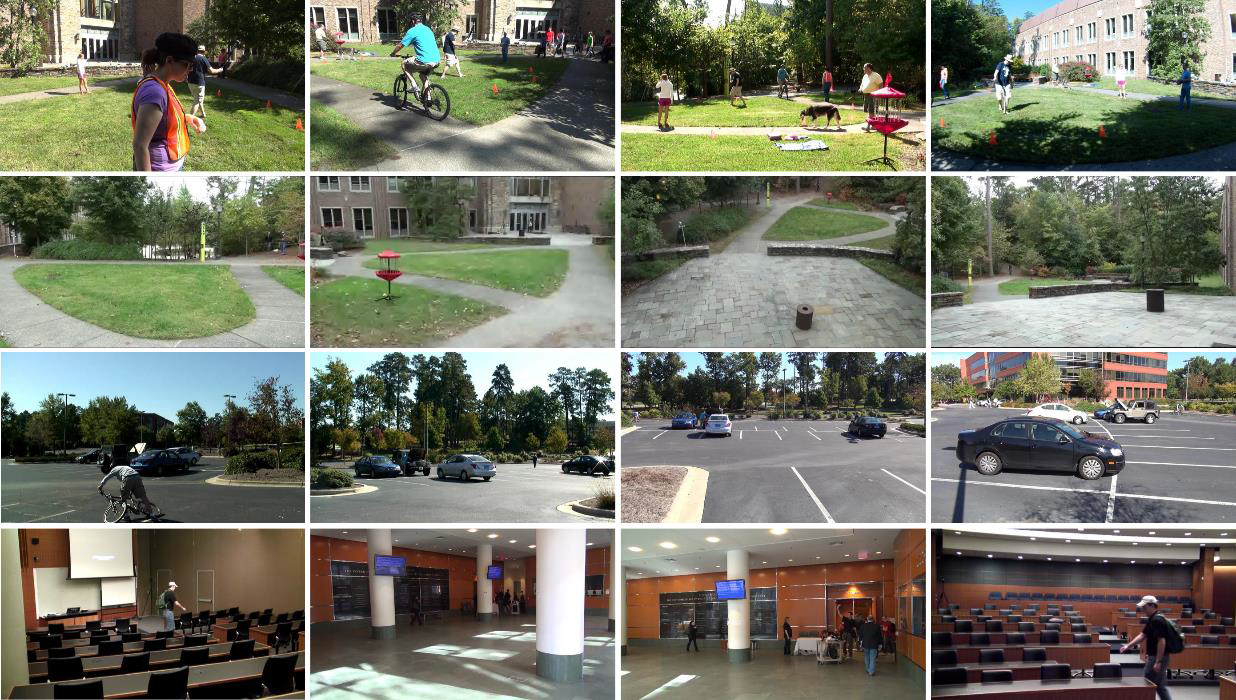
Reference
- [1] Cross-view People Tracking by Scene-centered Spatio-temporal Parsing, Yuanlu Xu, Xiaobai Liu, Lei Qin, Song-Chun Zhu. AAAI Conference on Artificial Intelligence (AAAI), 2017. [pdf]
- [2] Multi-view People Tracking via Hierarchical Trajectory Composition, Yuanlu Xu, Xiaobai Liu, Yang Liu, Song-Chun Zhu. In Proc. of IEEE Conference on Computer Vision and Pattern Recogntion (CVPR), 2016. [pdf]