Gender Bias in Contextualized Word Embeddings
Jieyu Zhao, Tianlu Wang, Mark Yatskar, Ryan Cotterell, Vicente Ordonez, and Kai-Wei Chang, in NAACL (short), 2019.
SlidesDownload the full text
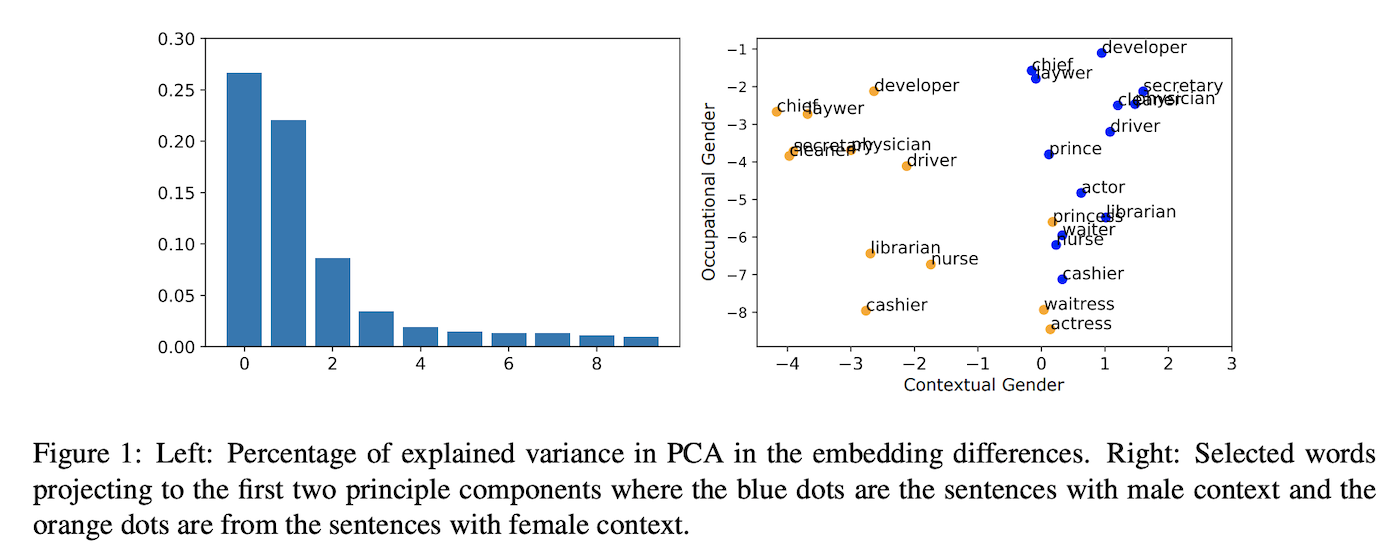
Abstract
Despite the great success of contextualized word embeddings on downstream applications, these representations potentially embed the societal biases exhibited in their training corpus. In this paper, we quantify, analyze and mitigate the gender bias exhibited in ELMo contextualized word vectors. We first demonstrate that the vectors encode and propagate information about genders unequally and then conduct a principal component analysis to visualize the geometry of the gender information in the embeddings. Then we show that ELMo works unequally well for men and women in down-stream tasks. Finally, we explore a variety of methods to remove such gender bias and demonstrate that it can be reduced through data augmentation.
Bib Entry
@inproceedings{zhao2019gender, author = {Zhao, Jieyu and Wang, Tianlu and Yatskar, Mark and Cotterell, Ryan and Ordonez, Vicente and Chang, Kai-Wei}, title = {Gender Bias in Contextualized Word Embeddings}, booktitle = {NAACL (short)}, year = {2019} }
Related Publications
-
Mitigating Gender Bias in Distilled Language Models via Counterfactual Role Reversal
Umang Gupta, Jwala Dhamala, Varun Kumar, Apurv Verma, Yada Pruksachatkun, Satyapriya Krishna, Rahul Gupta, Kai-Wei Chang, Greg Ver Steeg, and Aram Galstyan, in ACL Finding, 2022.
Full Text Abstract BibTeX DetailsLanguage models excel at generating coherent text, and model compression techniques such as knowledge distillation have enabled their use in resource-constrained settings. However, these models can be biased in multiple ways, including the unfounded association of male and female genders with gender-neutral professions. Therefore, knowledge distillation without any fairness constraints may preserve or exaggerate the teacher model’s biases onto the distilled model. To this end, we present a novel approach to mitigate gender disparity in text generation by learning a fair model during knowledge distillation. We propose two modifications to the base knowledge distillation based on counterfactual role reversal – modifying teacher probabilities and augmenting the training set. We evaluate gender polarity across professions in open-ended text generated from the resulting distilled and finetuned GPT-2models and demonstrate a substantial reduction in gender disparity with only a minor compromise in utility. Finally, we observe that language models that reduce gender polarity in language generation do not improve embedding fairness or downstream classification fairness.
@inproceedings{gupta2022equitable, title = {Mitigating Gender Bias in Distilled Language Models via Counterfactual Role Reversal}, author = {Gupta, Umang and Dhamala, Jwala and Kumar, Varun and Verma, Apurv and Pruksachatkun, Yada and Krishna, Satyapriya and Gupta, Rahul and Chang, Kai-Wei and Steeg, Greg Ver and Galstyan, Aram}, booktitle = {ACL Finding}, year = {2022} }
-
Harms of Gender Exclusivity and Challenges in Non-Binary Representation in Language Technologies
Sunipa Dev, Masoud Monajatipoor, Anaelia Ovalle, Arjun Subramonian, Jeff Phillips, and Kai-Wei Chang, in EMNLP, 2021.
Full Text Slides Poster Abstract BibTeX DetailsGender is widely discussed in the context of language tasks and when examining the stereotypes propagated by language models. However, current discussions primarily treat gender as binary, which can perpetuate harms such as the cyclical erasure of non-binary gender identities. These harms are driven by model and dataset biases, which are consequences of the non-recognition and lack of understanding of non-binary genders in society. In this paper, we explain the complexity of gender and language around it, and survey non-binary persons to understand harms associated with the treatment of gender as binary in English language technologies. We also detail how current language representations (e.g., GloVe, BERT) capture and perpetuate these harms and related challenges that need to be acknowledged and addressed for representations to equitably encode gender information.
@inproceedings{dev2021harms, title = {Harms of Gender Exclusivity and Challenges in Non-Binary Representation in Language Technologies}, author = {Dev, Sunipa and Monajatipoor, Masoud and Ovalle, Anaelia and Subramonian, Arjun and Phillips, Jeff and Chang, Kai-Wei}, presentation_id = {https://underline.io/events/192/sessions/7788/lecture/37320-harms-of-gender-exclusivity-and-challenges-in-non-binary-representation-in-language-technologies}, blog_url = {https://uclanlp.medium.com/harms-of-gender-exclusivity-and-challenges-in-non-binary-representation-in-language-technologies-5f89891b5aee}, booktitle = {EMNLP}, year = {2021} }
-
Gender Bias in Multilingual Embeddings and Cross-Lingual Transfer
Jieyu Zhao, Subhabrata Mukherjee, Saghar Hosseini, Kai-Wei Chang, and Ahmed Hassan Awadallah, in ACL, 2020.
Full Text Slides Video Abstract BibTeX DetailsMultilingual representations embed words from many languages into a single semantic space such that words with similar meanings are close to each other regardless of the language. These embeddings have been widely used in various settings, such as cross-lingual transfer, where a natural language processing (NLP) model trained on one language is deployed to another language. While the cross-lingual transfer techniques are powerful, they carry gender bias from the source to target languages. In this paper, we study gender bias in multilingual embeddings and how it affects transfer learning for NLP applications. We create a multilingual dataset for bias analysis and propose several ways for quantifying bias in multilingual representations from both the intrinsic and extrinsic perspectives. Experimental results show that the magnitude of bias in the multilingual representations changes differently when we align the embeddings to different target spaces and that the alignment direction can also have an influence on the bias in transfer learning. We further provide recommendations for using the multilingual word representations for downstream tasks.
@inproceedings{zhao2020gender, author = {Zhao, Jieyu and Mukherjee, Subhabrata and Hosseini, Saghar and Chang, Kai-Wei and Awadallah, Ahmed Hassan}, title = {Gender Bias in Multilingual Embeddings and Cross-Lingual Transfer}, booktitle = {ACL}, year = {2020}, presentation_id = {https://virtual.acl2020.org/paper_main.260.html} }
-
Examining Gender Bias in Languages with Grammatical Gender
Pei Zhou, Weijia Shi, Jieyu Zhao, Kuan-Hao Huang, Muhao Chen, Ryan Cotterell, and Kai-Wei Chang, in EMNLP, 2019.
Full Text Poster Code Abstract BibTeX DetailsRecent studies have shown that word embeddings exhibit gender bias inherited from the training corpora. However, most studies to date have focused on quantifying and mitigating such bias only in English. These analyses cannot be directly extended to languages that exhibit morphological agreement on gender, such as Spanish and French. In this paper, we propose new metrics for evaluating gender bias in word embeddings of these languages and further demonstrate evidence of gender bias in bilingual embeddings which align these languages with English. Finally, we extend an existing approach to mitigate gender bias in word embeddings under both monolingual and bilingual settings. Experiments on modified Word Embedding Association Test, word similarity, word translation, and word pair translation tasks show that the proposed approaches effectively reduce the gender bias while preserving the utility of the embeddings.
@inproceedings{zhou2019examining, author = {Zhou, Pei and Shi, Weijia and Zhao, Jieyu and Huang, Kuan-Hao and Chen, Muhao and Cotterell, Ryan and Chang, Kai-Wei}, title = {Examining Gender Bias in Languages with Grammatical Gender}, booktitle = {EMNLP}, year = {2019} }
-
Balanced Datasets Are Not Enough: Estimating and Mitigating Gender Bias in Deep Image Representations
Tianlu Wang, Jieyu Zhao, Mark Yatskar, Kai-Wei Chang, and Vicente Ordonez, in ICCV, 2019.
Full Text Code Demo Abstract BibTeX DetailsIn this work, we present a framework to measure and mitigate intrinsic biases with respect to protected variables –such as gender– in visual recognition tasks. We show that trained models significantly amplify the association of target labels with gender beyond what one would expect from biased datasets. Surprisingly, we show that even when datasets are balanced such that each label co-occurs equally with each gender, learned models amplify the association between labels and gender, as much as if data had not been balanced! To mitigate this, we adopt an adversarial approach to remove unwanted features corresponding to protected variables from intermediate representations in a deep neural network – and provide a detailed analysis of its effectiveness. Experiments on two datasets: the COCO dataset (objects), and the imSitu dataset (actions), show reductions in gender bias amplification while maintaining most of the accuracy of the original models.
@inproceedings{wang2019balanced, author = {Wang, Tianlu and Zhao, Jieyu and Yatskar, Mark and Chang, Kai-Wei and Ordonez, Vicente}, title = {Balanced Datasets Are Not Enough: Estimating and Mitigating Gender Bias in Deep Image Representations}, booktitle = {ICCV}, year = {2019} }
-
Gender Bias in Contextualized Word Embeddings
Jieyu Zhao, Tianlu Wang, Mark Yatskar, Ryan Cotterell, Vicente Ordonez, and Kai-Wei Chang, in NAACL (short), 2019.
Full Text Slides Video Abstract BibTeX DetailsDespite the great success of contextualized word embeddings on downstream applications, these representations potentially embed the societal biases exhibited in their training corpus. In this paper, we quantify, analyze and mitigate the gender bias exhibited in ELMo contextualized word vectors. We first demonstrate that the vectors encode and propagate information about genders unequally and then conduct a principal component analysis to visualize the geometry of the gender information in the embeddings. Then we show that ELMo works unequally well for men and women in down-stream tasks. Finally, we explore a variety of methods to remove such gender bias and demonstrate that it can be reduced through data augmentation.
@inproceedings{zhao2019gender, author = {Zhao, Jieyu and Wang, Tianlu and Yatskar, Mark and Cotterell, Ryan and Ordonez, Vicente and Chang, Kai-Wei}, title = {Gender Bias in Contextualized Word Embeddings}, booktitle = {NAACL (short)}, year = {2019} }
-
Learning Gender-Neutral Word Embeddings
Jieyu Zhao, Yichao Zhou, Zeyu Li, Wei Wang, and Kai-Wei Chang, in EMNLP (short), 2018.
Full Text Code Abstract BibTeX DetailsWord embeddings have become a fundamental component in a wide range of Natu-ral Language Processing (NLP) applications.However, these word embeddings trained onhuman-generated corpora inherit strong gen-der stereotypes that reflect social constructs.In this paper, we propose a novel word em-bedding model, De-GloVe, that preserves gen-der information in certain dimensions of wordvectors while compelling other dimensions tobe free of gender influence. Quantitative andqualitative experiments demonstrate that De-GloVe successfully isolates gender informa-tion without sacrificing the functionality of theembedding model.
@inproceedings{zhao2018learning, author = {Zhao, Jieyu and Zhou, Yichao and Li, Zeyu and Wang, Wei and Chang, Kai-Wei}, title = {Learning Gender-Neutral Word Embeddings}, booktitle = {EMNLP (short)}, year = {2018} }
-
Man is to Computer Programmer as Woman is to Homemaker? Debiasing Word Embeddings
Tolga Bolukbasi, Kai-Wei Chang, James Zou, Venkatesh Saligrama, and Adam Kalai, in NeurIPS, 2016.
Full Text Code Abstract BibTeX Details Top-10 cited paper at NeurIPS 16The blind application of machine learning runs the risk of amplifying biases present in data. Such a danger is facing us with word embedding, a popular framework to represent text data as vectors which has been used in many machine learning and natural language processing tasks. We show that even word embeddings trained on Google News articles exhibit female/male gender stereotypes to a disturbing extent. This raises concerns because their widespread use, as we describe, often tends to amplify these biases. Geometrically, gender bias is first shown to be captured by a direction in the word embedding. Second, gender neutral words are shown to be linearly separable from gender definition words in the word embedding. Using these properties, we provide a methodology for modifying an embedding to remove gender stereotypes, such as the association between between the words receptionist and female, while maintaining desired associations such as between the words queen and female. We define metrics to quantify both direct and indirect gender biases in embeddings, and develop algorithms to "debias" the embedding. Using crowd-worker evaluation as well as standard benchmarks, we empirically demonstrate that our algorithms significantly reduce gender bias in embeddings while preserving the its useful properties such as the ability to cluster related concepts and to solve analogy tasks. The resulting embeddings can be used in applications without amplifying gender bias.
@inproceedings{bolukbasi2016man, author = {Bolukbasi, Tolga and Chang, Kai-Wei and Zou, James and Saligrama, Venkatesh and Kalai, Adam}, title = {Man is to Computer Programmer as Woman is to Homemaker? Debiasing Word Embeddings}, booktitle = {NeurIPS}, year = {2016} }