Robustness Verification for Transformers
Zhouxing Shi, Huan Zhang, Kai-Wei Chang, Minlie Huang, and Cho-Jui Hsieh, in ICLR, 2020.
CodeDownload the full text
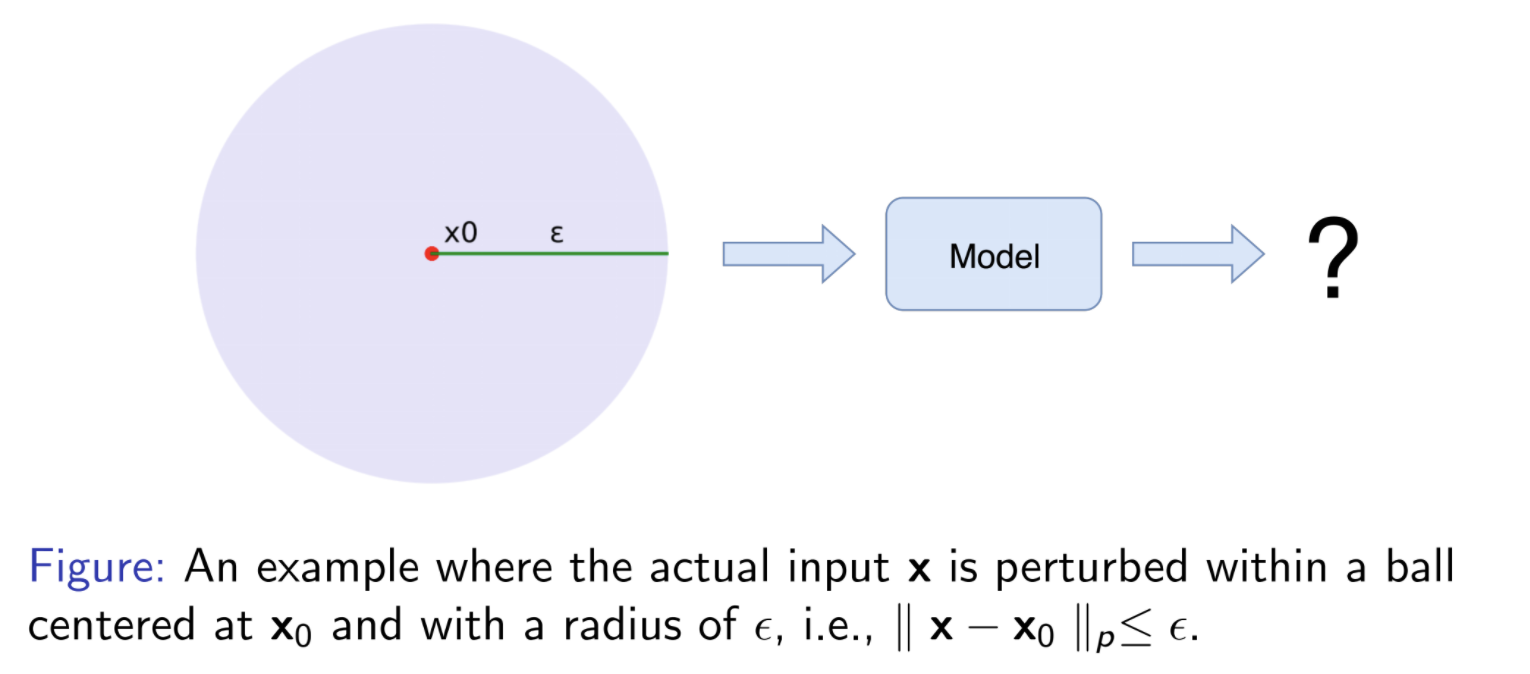
Abstract
Robustness verification that aims to formally certify the prediction behavior of neural networks has become an important tool for understanding the behavior of a given model and for obtaining safety guarantees. However, previous methods are usually limited to relatively simple neural networks. In this paper, we consider the robustness verification problem for Transformers. Transformers have complex self-attention layers that pose many challenges for verification, including cross-nonlinearity and cross-position dependency, which have not been discussed in previous work. We resolve these challenges and develop the first verification algorithm for Transformers. The certified robustness bounds computed by our method are significantly tighter than those by naive Interval Bound Propagation. These bounds also shed light on interpreting Transformers as they consistently reflect the importance of words in sentiment analysis.
Our #ICLR2020 paper, Robustness Verification for Transformers, presents the first algorithm for verifying Transformers (w/ Huan Zhang, @kaiwei_chang, Minlie Huang and Cho-Jui Hsieh) . It's available at https://t.co/1tXyBVKSnS and https://t.co/Q4fc5cnlZ3.
— Zhouxing Shi (@zhouxingshi) February 18, 2020
Bib Entry
@inproceedings{shi2020robustness, author = {Shi, Zhouxing and Zhang, Huan and Chang, Kai-Wei and Huang, Minlie and Hsieh, Cho-Jui}, title = {Robustness Verification for Transformers}, booktitle = {ICLR}, year = {2020} }
Related Publications
-
VideoCon: Robust video-language alignment via contrast captions
Hritik Bansal, Yonatan Bitton, Idan Szpektor, Kai-Wei Chang, and Aditya Grover, in CVPR, 2024.
Full Text Code Demo Abstract BibTeX DetailsDespite being (pre)trained on a massive amount of data, state-of-the-art video-language alignment models are not robust to semantically-plausible contrastive changes in the video captions. Our work addresses this by identifying a broad spectrum of contrast misalignments, such as replacing entities, actions, and flipping event order, which alignment models should be robust against. To this end, we introduce the VideoCon, a video-language alignment dataset constructed by a large language model that generates plausible contrast video captions and explanations for differences between original and contrast video captions. Then, a generative video-language model is finetuned with VideoCon to assess video-language entailment and generate explanations. Our VideoCon-based alignment model significantly outperforms current models. It exhibits a 12-point increase in AUC for the video-language alignment task on human-generated contrast captions. Finally, our model sets new state of the art zero-shot performance in temporally-extensive video-language tasks such as text-to-video retrieval (SSv2-Temporal) and video question answering (ATP-Hard). Moreover, our model shows superior performance on novel videos and human-crafted captions and explanations.
@inproceedings{bansal2023videocon, author = {Bansal, Hritik and Bitton, Yonatan and Szpektor, Idan and Chang, Kai-Wei and Grover, Aditya}, title = {VideoCon: Robust video-language alignment via contrast captions}, booktitle = {CVPR}, year = {2024} }
-
Red Teaming Language Model Detectors with Language Models
Zhouxing Shi, Yihan Wang, Fan Yin, Xiangning Chen, Kai-Wei Chang, and Cho-Jui Hsieh, in TACL, 2023.
Full Text Code Abstract BibTeX DetailsThe prevalence and high capacity of large language models (LLMs) present significant safety and ethical risks when malicious users exploit them for automated content generation. To prevent the potentially deceptive usage of LLMs, recent works have proposed several algorithms to detect machine-generated text. In this paper, we systematically test the reliability of the existing detectors, by designing two types of attack strategies to fool the detectors: 1) replacing words with their synonyms based on the context; 2) altering the writing style of generated text. These strategies are implemented by instructing LLMs to generate synonymous word substitutions or writing directives that modify the style without human involvement, and the LLMs leveraged in the attack can also be protected by detectors. Our research reveals that our attacks effectively compromise the performance of all tested detectors, thereby underscoring the urgent need for the development of more robust machine-generated text detection systems.
@inproceedings{shi2023red, author = {Shi, Zhouxing and Wang, Yihan and Yin, Fan and Chen, Xiangning and Chang, Kai-Wei and Hsieh, Cho-Jui}, title = {Red Teaming Language Model Detectors with Language Models}, booktitle = {TACL}, year = {2023} }
-
CleanCLIP: Mitigating Data Poisoning Attacks in Multimodal Contrastive Learning
Hritik Bansal, Nishad Singhi, Yu Yang, Fan Yin, Aditya Grover, and Kai-Wei Chang, in ICCV, 2023.
Full Text Code BibTeX Details Best Paper Award at ICLR Workshop, Oral at ICCV (195 out of 8088 submissions, top 2.5%)@inproceedings{bansal2023cleanclip, author = {Bansal, Hritik and Singhi, Nishad and Yang, Yu and Yin, Fan and Grover, Aditya and Chang, Kai-Wei}, title = {CleanCLIP: Mitigating Data Poisoning Attacks in Multimodal Contrastive Learning}, booktitle = {ICCV}, year = {2023}, https://openreview.net/pdf?id = gfgcnevrfhv }
-
ADDMU: Detection of Far-Boundary Adversarial Examples with Data and Model Uncertainty Estimation
Fan Yin, Yao Li, Cho-Jui Hsieh, and Kai-Wei Chang, in EMNLP, 2022.
Full Text Abstract BibTeX DetailsAdversarial Examples Detection (AED) is a crucial defense technique against adversarial attacks and has drawn increasing attention from the Natural Language Processing (NLP) community. Despite the surge of new AED methods, our studies show that existing methods heavily rely on a shortcut to achieve good performance. In other words, current search-based adversarial attacks in NLP stop once model predictions change, and thus most adversarial examples generated by those attacks are located near model decision boundaries. To surpass this shortcut and fairly evaluate AED methods, we propose to test AED methods with Far Boundary (FB) adversarial examples. Existing methods show worse than random guess performance under this scenario. To overcome this limitation, we propose a new technique, ADDMU, adversary detection with data and model uncertainty, which combines two types of uncertainty estimation for both regular and FB adversarial example detection. Our new method outperforms previous methods by 3.6 and 6.0 AUC points under each scenario. Finally, our analysis shows that the two types of uncertainty provided by ADDMU can be leveraged to characterize adversarial examples and identify the ones that contribute most to model’s robustness in adversarial training.
@inproceedings{yin2022addmu, title = {ADDMU: Detection of Far-Boundary Adversarial Examples with Data and Model Uncertainty Estimation}, author = {Yin, Fan and Li, Yao and Hsieh, Cho-Jui and Chang, Kai-Wei}, booktitle = {EMNLP}, year = {2022} }
-
Investigating Ensemble Methods for Model Robustness Improvement of Text Classifiers
Jieyu Zhao, Xuezhi Wang, Yao Qin, Jilin Chen, and Kai-Wei Chang, in EMNLP-Finding (short), 2022.
Full Text BibTeX Details@inproceedings{zhao2022investigating, title = { Investigating Ensemble Methods for Model Robustness Improvement of Text Classifiers}, author = {Zhao, Jieyu and Wang, Xuezhi and Qin, Yao and Chen, Jilin and Chang, Kai-Wei}, booktitle = {EMNLP-Finding (short)}, year = {2022} }
-
Unsupervised Syntactically Controlled Paraphrase Generation with Abstract Meaning Representations
Kuan-Hao Huang, Varun Iyer, Anoop Kumar, Sriram Venkatapathy, Kai-Wei Chang, and Aram Galstyan, in EMNLP-Finding (short), 2022.
Full Text BibTeX Details@inproceedings{huang2022unsupervised, title = {Unsupervised Syntactically Controlled Paraphrase Generation with Abstract Meaning Representations}, author = {Huang, Kuan-Hao and Iyer, Varun and Kumar, Anoop and Venkatapathy, Sriram and Chang, Kai-Wei and Galstyan, Aram}, booktitle = {EMNLP-Finding (short)}, year = {2022} }
-
Improving the Adversarial Robustness of NLP Models by Information Bottleneck
Cenyuan Zhang, Xiang Zhou, Yixin Wan, Xiaoqing Zheng, Kai-Wei Chang, and Cho-Jui Hsieh, in ACL-Finding, 2022.
Full Text Abstract BibTeX DetailsExisting studies have demonstrated that adversarial examples can be directly attributed to the presence of non-robust features, which are highly predictive, but can be easily manipulated by adversaries to fool NLP models. In this study, we explore the feasibility of capturing task-specific robust features, while eliminating the non-robust ones by using the information bottleneck theory. Through extensive experiments, we show that the models trained with our information bottleneck-based method are able to achieve a significant improvement in robust accuracy, exceeding performances of all the previously reported defense methods while suffering almost no performance drop in clean accuracy on SST-2, AGNEWS and IMDB datasets.
@inproceedings{zhang2022improving, title = {Improving the Adversarial Robustness of NLP Models by Information Bottleneck}, author = {Zhang, Cenyuan and Zhou, Xiang and Wan, Yixin and Zheng, Xiaoqing and Chang, Kai-Wei and Hsieh, Cho-Jui}, booktitle = {ACL-Finding}, year = {2022} }
-
Searching for an Effiective Defender: Benchmarking Defense against Adversarial Word Substitution
Zongyi Li, Jianhan Xu, Jiehang Zeng, Linyang Li, Xiaoqing Zheng, Qi Zhang, Kai-Wei Chang, and Cho-Jui Hsieh, in EMNLP, 2021.
Full Text Abstract BibTeX DetailsRecent studies have shown that deep neural networks are vulnerable to intentionally crafted adversarial examples, and various methods have been proposed to defend against adversarial word-substitution attacks for neural NLP models. However, there is a lack of systematic study on comparing different defense approaches under the same attacking setting. In this paper, we seek to fill the gap of systematic studies through comprehensive researches on understanding the behavior of neural text classifiers trained by various defense methods under representative adversarial attacks. In addition, we propose an effective method to further improve the robustness of neural text classifiers against such attacks and achieved the highest accuracy on both clean and adversarial examples on AGNEWS and IMDB datasets by a significant margin.
@inproceedings{li2021searching, title = {Searching for an Effiective Defender: Benchmarking Defense against Adversarial Word Substitution}, author = {Li, Zongyi and Xu, Jianhan and Zeng, Jiehang and Li, Linyang and Zheng, Xiaoqing and Zhang, Qi and Chang, Kai-Wei and Hsieh, Cho-Jui}, presentation_id = {https://underline.io/events/192/posters/8225/poster/38025-searching-for-an-effective-defender-benchmarking-defense-against-adversarial-word-substitution}, booktitle = {EMNLP}, year = {2021} }
-
On the Transferability of Adversarial Attacks against Neural Text Classifier
Liping Yuan, Xiaoqing Zheng, Yi Zhou, Cho-Jui Hsieh, and Kai-Wei Chang, in EMNLP, 2021.
Full Text Abstract BibTeX DetailsDeep neural networks are vulnerable to adversarial attacks, where a small perturbation to an input alters the model prediction. In many cases, malicious inputs intentionally crafted for one model can fool another model. In this paper, we present the first study to systematically investigate the transferability of adversarial examples for text classification models and explore how various factors, including network architecture, tokenization scheme, word embedding, and model capacity, affect the transferability of adversarial examples. Based on these studies, we propose a genetic algorithm to find an ensemble of models that can be used to induce adversarial examples to fool almost all existing models. Such adversarial examples reflect the defects of the learning process and the data bias in the training set. Finally, we derive word replacement rules that can be used for model diagnostics from these adversarial examples.
@inproceedings{yuan2021on, title = {On the Transferability of Adversarial Attacks against Neural Text Classifier}, author = {Yuan, Liping and Zheng, Xiaoqing and Zhou, Yi and Hsieh, Cho-Jui and Chang, Kai-Wei}, presentation_id = {https://underline.io/events/192/posters/8223/poster/38067-on-the-transferability-of-adversarial-attacks-against-neural-text-classifier}, booktitle = {EMNLP}, year = {2021} }
-
Defense against Synonym Substitution-based Adversarial Attacks via Dirichlet Neighborhood Ensemble
Yi Zhou, Xiaoqing Zheng, Cho-Jui Hsieh, Kai-Wei Chang, and Xuanjing Huang, in ACL, 2021.
Full Text Code Abstract BibTeX DetailsAlthough deep neural networks have achieved prominent performance on many NLP tasks, they are vulnerable to adversarial examples. We propose Dirichlet Neighborhood Ensemble (DNE), a randomized method for training a robust model to defense synonym substitutionbased attacks. During training, DNE forms virtual sentences by sampling embedding vectors for each word in an input sentence from a convex hull spanned by the word and its synonyms, and it augments them with the training data. In such a way, the model is robust to adversarial attacks while maintaining the performance on the original clean data. DNE is agnostic to the network architectures and scales to large models (e.g., BERT) for NLP applications. Through extensive experimentation, we demonstrate that our method consistently outperforms recently proposed defense methods by a significant margin across different network architectures and multiple data sets.
@inproceedings{zhou2021defense, title = {Defense against Synonym Substitution-based Adversarial Attacks via Dirichlet Neighborhood Ensemble}, author = {Zhou, Yi and Zheng, Xiaoqing and Hsieh, Cho-Jui and Chang, Kai-Wei and Huang, Xuanjing}, booktitle = {ACL}, year = {2021} }
-
Double Perturbation: On the Robustness of Robustness and Counterfactual Bias Evaluation
Chong Zhang, Jieyu Zhao, Huan Zhang, Kai-Wei Chang, and Cho-Jui Hsieh, in NAACL, 2021.
Full Text Video Code Abstract BibTeX DetailsRobustness and counterfactual bias are usually evaluated on a test dataset. However, are these evaluations robust? If the test dataset is perturbed slightly, will the evaluation results keep the same? In this paper, we propose a "double perturbation" framework to uncover model weaknesses beyond the test dataset. The framework first perturbs the test dataset to construct abundant natural sentences similar to the test data, and then diagnoses the prediction change regarding a single-word substitution. We apply this framework to study two perturbation-based approaches that are used to analyze models’ robustness and counterfactual bias in English. (1) For robustness, we focus on synonym substitutions and identify vulnerable examples where prediction can be altered. Our proposed attack attains high success rates (96.0%-99.8%) in finding vulnerable examples on both original and robustly trained CNNs and Transformers. (2) For counterfactual bias, we focus on substituting demographic tokens (e.g., gender, race) and measure the shift of the expected prediction among constructed sentences. Our method is able to reveal the hidden model biases not directly shown in the test dataset.
@inproceedings{zhang2021double, title = { Double Perturbation: On the Robustness of Robustness and Counterfactual Bias Evaluation}, booktitle = {NAACL}, author = {Zhang, Chong and Zhao, Jieyu and Zhang, Huan and Chang, Kai-Wei and Hsieh, Cho-Jui}, year = {2021}, presentation_id = {https://underline.io/events/122/sessions/4229/lecture/19609-double-perturbation-on-the-robustness-of-robustness-and-counterfactual-bias-evaluation} }
-
Provable, Scalable and Automatic Perturbation Analysis on General Computational Graphs
Kaidi Xu, Zhouxing Shi, Huan Zhang, Yihan Wang, Kai-Wei Chang, Minlie Huang, Bhavya Kailkhura, Xue Lin, and Cho-Jui Hsieh, in NeurIPS, 2020.
Full Text Code Abstract BibTeX DetailsLinear relaxation based perturbation analysis (LiRPA) for neural networks, which computes provable linear bounds of output neurons given a certain amount of input perturbation, has become a core component in robustness verification and certified defense. The majority of LiRPA-based methods only consider simple feed-forward networks and it needs particular manual derivations and implementations when extended to other architectures. In this paper, we develop an automatic framework to enable perturbation analysis on any neural network structures, by generalizing exiting LiRPA algorithms such as CROWN to operate on general computational graphs. The flexibility, differentiability and ease of use of our framework allow us to obtain state-of-the-art results on LiRPA based certified defense on fairly complicated networks like DenseNet, ResNeXt and Transformer that are not supported by prior work. Our framework also enables loss fusion, a technique that significantly reduces the computational complexity of LiRPA for certified defense. For the first time, we demonstrate LiRPA based certified defense on Tiny ImageNet and Downscaled ImageNet where previous approaches cannot scale to due to the relatively large number of classes. Our work also yields an open-source library for the community to apply LiRPA to areas beyond certified defense without much LiRPA expertise, e.g., we create a neural network with a provably flat optimization landscape. Our open source library is available at https://github.com/KaidiXu/auto_LiRPA
@inproceedings{xu2020provable, author = {Xu, Kaidi and Shi, Zhouxing and Zhang, Huan and Wang, Yihan and Chang, Kai-Wei and Huang, Minlie and Kailkhura, Bhavya and Lin, Xue and Hsieh, Cho-Jui}, title = {Provable, Scalable and Automatic Perturbation Analysis on General Computational Graphs}, booktitle = {NeurIPS}, year = {2020} }
-
On the Robustness of Language Encoders against Grammatical Errors
Fan Yin, Quanyu Long, Tao Meng, and Kai-Wei Chang, in ACL, 2020.
Full Text Slides Video Code Abstract BibTeX DetailsWe conduct a thorough study to diagnose the behaviors of pre-trained language encoders (ELMo, BERT, and RoBERTa) when confronted with natural grammatical errors. Specifically, we collect real grammatical errors from non-native speakers and conduct adversarial attacks to simulate these errors on clean text data. We use this approach to facilitate debugging models on downstream applications. Results confirm that the performance of all tested models is affected but the degree of impact varies. To interpret model behaviors, we further design a linguistic acceptability task to reveal their abilities in identifying ungrammatical sentences and the position of errors. We find that fixed contextual encoders with a simple classifier trained on the prediction of sentence correctness are able to locate error positions. We also design a cloze test for BERT and discover that BERT captures the interaction between errors and specific tokens in context. Our results shed light on understanding the robustness and behaviors of language encoders against grammatical errors.
@inproceedings{yin2020robustness, author = {Yin, Fan and Long, Quanyu and Meng, Tao and Chang, Kai-Wei}, title = {On the Robustness of Language Encoders against Grammatical Errors}, booktitle = {ACL}, presentation_id = {https://virtual.acl2020.org/paper_main.310.html}, year = {2020} }
-
Robustness Verification for Transformers
Zhouxing Shi, Huan Zhang, Kai-Wei Chang, Minlie Huang, and Cho-Jui Hsieh, in ICLR, 2020.
Full Text Video Code Abstract BibTeX DetailsRobustness verification that aims to formally certify the prediction behavior of neural networks has become an important tool for understanding the behavior of a given model and for obtaining safety guarantees. However, previous methods are usually limited to relatively simple neural networks. In this paper, we consider the robustness verification problem for Transformers. Transformers have complex self-attention layers that pose many challenges for verification, including cross-nonlinearity and cross-position dependency, which have not been discussed in previous work. We resolve these challenges and develop the first verification algorithm for Transformers. The certified robustness bounds computed by our method are significantly tighter than those by naive Interval Bound Propagation. These bounds also shed light on interpreting Transformers as they consistently reflect the importance of words in sentiment analysis.
@inproceedings{shi2020robustness, author = {Shi, Zhouxing and Zhang, Huan and Chang, Kai-Wei and Huang, Minlie and Hsieh, Cho-Jui}, title = {Robustness Verification for Transformers}, booktitle = {ICLR}, year = {2020} }
-
Learning to Discriminate Perturbations for Blocking Adversarial Attacks in Text Classification
Yichao Zhou, Jyun-Yu Jiang, Kai-Wei Chang, and Wei Wang, in EMNLP, 2019.
Full Text Code Abstract BibTeX DetailsAdversarial attacks against machine learning models have threatened various real-world applications such as spam filtering and sentiment analysis. In this paper, we propose a novel framework, learning to DIScriminate Perturbations (DISP), to identify and adjust malicious perturbations, thereby blocking adversarial attacks for text classification models. To identify adversarial attacks, a perturbation discriminator validates how likely a token in the text is perturbed and provides a set of potential perturbations. For each potential perturbation, an embedding estimator learns to restore the embedding of the original word based on the context and a replacement token is chosen based on approximate kNN search. DISP can block adversarial attacks for any NLP model without modifying the model structure or training procedure. Extensive experiments on two benchmark datasets demonstrate that DISP significantly outperforms baseline methods in blocking adversarial attacks for text classification. In addition, in-depth analysis shows the robustness of DISP across different situations.
@inproceedings{zhou2019learning, author = {Zhou, Yichao and Jiang, Jyun-Yu and Chang, Kai-Wei and Wang, Wei}, title = {Learning to Discriminate Perturbations for Blocking Adversarial Attacks in Text Classification}, booktitle = {EMNLP}, year = {2019} }
-
Retrofitting Contextualized Word Embeddings with Paraphrases
Weijia Shi, Muhao Chen, Pei Zhou, and Kai-Wei Chang, in EMNLP (short), 2019.
Full Text Slides Video Code Abstract BibTeX DetailsContextualized word embedding models, such as ELMo, generate meaningful representations of words and their context. These models have been shown to have a great impact on downstream applications. However, in many cases, the contextualized embedding of a word changes drastically when the context is paraphrased. As a result, the downstream model is not robust to paraphrasing and other linguistic variations. To enhance the stability of contextualized word embedding models, we propose an approach to retrofitting contextualized embedding models with paraphrase contexts. Our method learns an orthogonal transformation on the input space, which seeks to minimize the variance of word representations on paraphrased contexts. Experiments show that the retrofitted model significantly outperforms the original ELMo on various sentence classification and language inference tasks.
@inproceedings{shi2019retrofitting, author = {Shi, Weijia and Chen, Muhao and Zhou, Pei and Chang, Kai-Wei}, title = {Retrofitting Contextualized Word Embeddings with Paraphrases}, booktitle = {EMNLP (short)}, vimeo_id = {430797636}, year = {2019} }
-
Generating Natural Language Adversarial Examples
Moustafa Alzantot, Yash Sharma, Ahmed Elgohary, Bo-Jhang Ho, Mani Srivastava, and Kai-Wei Chang, in EMNLP (short), 2018.
Full Text Code Abstract BibTeX Details Top-10 cited paper at EMNLP 18Deep neural networks (DNNs) are vulnerable to adversarial examples, perturbations to correctly classified examples which can cause the network to misclassify. In the image domain, these perturbations can often be made virtually indistinguishable to human perception, causing humans and state-of-the-art models to disagree. However, in the natural language domain, small perturbations are clearly perceptible, and the replacement of a single word can drastically alter the semantics of the document. Given these challenges, we use a population-based optimization algorithm to generate semantically and syntactically similar adversarial examples. We demonstrate via a human study that 94.3% of the generated examples are classified to the original label by human evaluators, and that the examples are perceptibly quite similar. We hope our findings encourage researchers to pursue improving the robustness of DNNs in the natural language domain.
@inproceedings{alzanto2018generating, author = {Alzantot, Moustafa and Sharma, Yash and Elgohary, Ahmed and Ho, Bo-Jhang and Srivastava, Mani and Chang, Kai-Wei}, title = {Generating Natural Language Adversarial Examples}, booktitle = {EMNLP (short)}, year = {2018} }